How AI on Edge Is Changing the Infrastructure of Smart Cities and Urban Mobility Projects
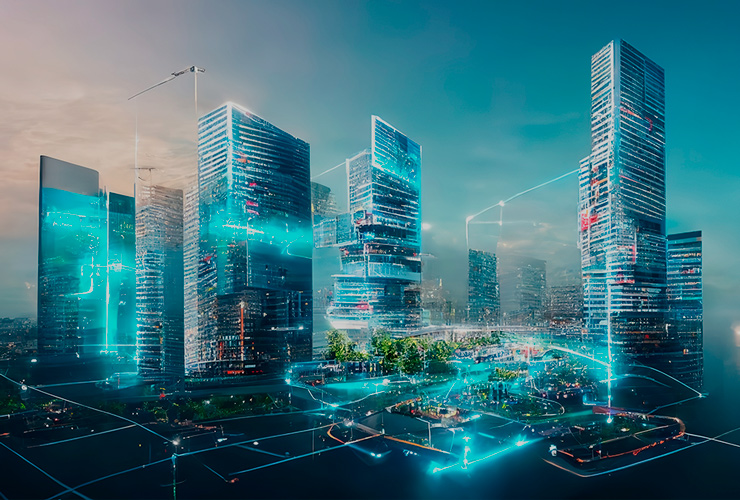
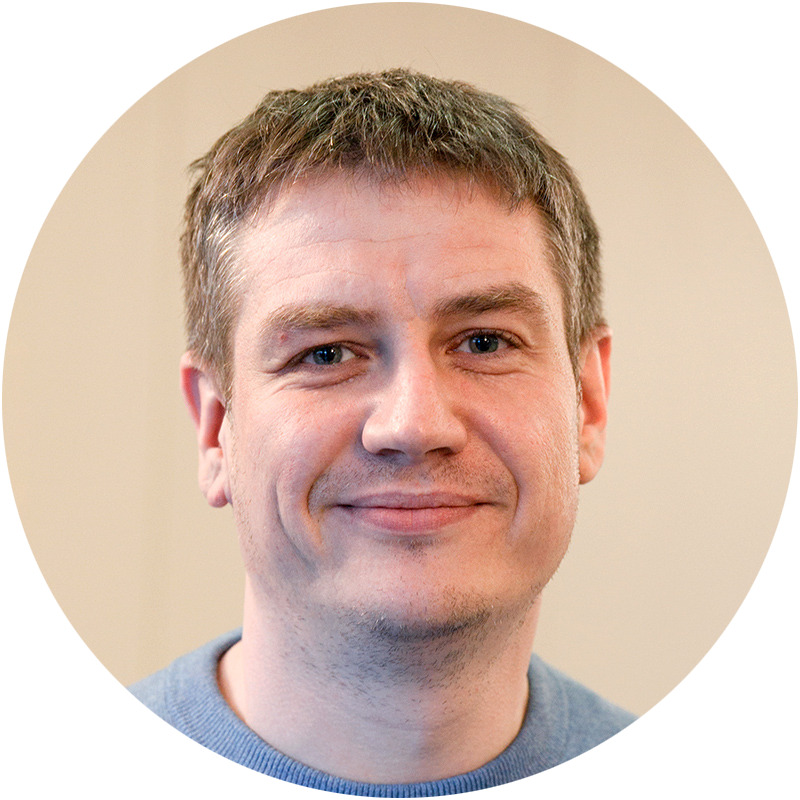
The AI and Edge Computing market is growing year by year, and this technology is entering people's everyday lives. In this article, we will demonstrate how the Promwad team develops AI-based solutions for our clients and how they can be applied in urban mobility, automotive, video surveillance, and other fields.
Table of contents
Edge AI: What It Is and What Its Benefits Are
How AI on Edge Is Changing Smart Cities and Urban Mobility Projects
How AI on Edge Can Be Used in Different Industries
Edge AI: What It Is and What Its Benefits Are
The Edge AI system consists of AI software algorithms and hardware. Recently, technologies in both components have reached a new level, opening up new horizons for their use in real applications.
AI algorithms are based on the use of mathematical models of neural networks and the learning process on large sets of labelled data. The theory and application framework of artificial intelligence are well-developed. Today, there are many academic and private companies developing and improving neural network models, collecting datasets, and creating tools for their labelling.
Most of these results are available as open-source code for engineers.
The hardware of such systems includes sensors to record data (such as audio or video signals), a computational module to run the neural network model, and an interface to transmit the results to the network for subsequent analysis and use in applications.
Today's microelectronic technologies make it possible to create ultra-low-cost processors with low power consumption and high computing performance: system on a chip (SoC), system on a module (SoM), and field-programmable gate array (FPGA). With these processors, it is possible to register and process data directly on the device without sending large amounts of numbers to the cloud for processing by powerful servers.
All of this has the following advantages:
- AI can be integrated with the sensor and housed in a small form factor.
- Massive video and audio files can be processed locally without being sent to the cloud, which speeds up system response. This feature is important for real-time applications such as ADAS in the automotive industry.
- The flow of data over the network is reduced, and it is possible to equip AI with more systems that send data processing results for high-level analysis to the cloud.
Data from multiple distributed devices can be further processed by machine learning methods. They identify patterns in the data structure, time and place of its registration and, with the help of statistical processing and optimisation algorithms, produce optimal solutions.
This makes it possible to apply AI and edge computing solutions to automatic transportation management, optimise fleet and trucking resources, and manage urban infrastructure.
How AI on Edge Is Changing Smart Cities and Urban Mobility Projects
By smart city and urban mobility projects, we mean a huge set of devices and systems that make living and moving around the city more convenient and safer. Here are just a few examples:
- Apps and charging stations for personal and shared electric cars and scooters. Driver assistance systems and smart parking.
- Smart video surveillance systems for the security and automation of shopping centres, construction sites, offices, and residences.
Combining AI algorithms with local computing gives us new market opportunities for comfortable driving, trucking, traffic, and fleet management. The development of new urban mobility projects transforms the infrastructure of smart cities to ensure the safety of drivers and pedestrians and to optimise urban space.
Here is a description of the usual software and hardware platform development process for smart city and urban mobility projects (we also mention typical market players here):
- Academic institutes and universities create neural network models (NNM) optimised for specific tasks: detection, classification, and object segmentation.
- Engineering companies, such as Brodmann17, purposefully collect data sets in a specific subject area and train NN models.
- Technology vendors, such as Ambarella, Analog Devices, Nvidia, and NXP, design chips, cameras, and computing platforms to record and process audio and video and to run AI algorithms.
- Software and hardware developers, such as Promwad, port finished NNs to devices, provide their local operation (AI on Edge) and, if necessary, train them to solve specific tasks.
- Manufacturers and integrators begin mass production and marketing of AI devices.
Our company takes its place in this chain as an independent design centre that helps our clients create custom software and hardware solutions for AI-based systems. Below, we will show how these projects become part of a person's everyday life and how our developments help high-tech companies enter the market with new breakthrough products.
Case Studies
Machine learning in the automotive industry: we are testing the system to estimate head position. The solution captures key points on the face, analyses the position of the head, and eyes, and thus "recognizes" sleepiness, sickness, or inattention.
Driver Head Tracking
We have designed a computer vision system to estimate head position and analyse driver fatigue, commissioned by a European engineering company. This solution, based on an off-the-shelf module with Ambarella chips, aids in the detection of dangerous conditions through the use of several indicators, including head movements, blink rate, and body position.
With ML, we can analyse the driver's emotions (high or low spirit) — tracking face landmarks relations, eye reactions and pose to predict their weariness and behaviour.
In addition to tracking a person's condition, AI on Edge solutions are used in ADAS development and other complex driver assistance systems. First of all, these are "smart" cameras that analyse the situation on the road while driving and help the driver avoid accidents. With the help of these solutions, it is possible to implement:
- full-speed-range adaptive cruise control;
- adaptive headlamp control;
- traffic sign recognition;
- forward collision warning;
- pedestrian detection and automatic braking;
- road quality assessment.
Automotive solutions based on AI on Edge work in real-time and help prevent dangerous situations, as information processing takes a tenth of a second.
AI algorithms ensure the privacy of personal data: after local processing, it is no longer photos or videos that are sent to the server but digitised results of analysis without the possibility of returning to the original view to obtain personal data.
Additional application possibilities for AI in the automotive industry — sensor fusion: the system combines camera, radar and lidar data to achieve greater accuracy and full 3D content. The radar captures speed, the lidar captures distance, and the NN information from the camera helps classify the object. Here's what data the system can capture and analyse:
- precise 3D object detection;
- range accuracy;
- free-space detection;
- object classification;
- object angular position;
- scene context.
Sensor fusion can be used in logistics to estimate the volume of bulk goods.
Complex solutions based on cameras, radars, and lidars can be used in logistics and construction. For example, they evaluate the volume of bulk cargo or help design manoeuvre systems for large vehicles or construction cranes.
Additional application possibilities for AI in video surveillance: cameras that help assess the condition of the driver can also be used in production to monitor the condition of workers. Face, object, and voice recognition systems assist in organising the admission system or controlling access to protected objects or residential complexes.
"Smart" bike parking based on an AI-powered camera by SONY: the solution designed at Promwad detects bicycles and captures available spaces. The camera can help solve the parking problem in tourist cities.
Smart Bike Parking
Promwad has designed a smart bicycle parking solution based on the SONY Spresense AI computer vision system. At the heart of this smart parking is a tracking system with the MobileNet neural network and the Tensorflow framework. It works as follows:
- reacts to human motion, turns on the light and power;
- automatically detects available bike parking spaces;
- and sends the information on parking availability over the GSM to the cloud.
This is the first system on the market developed on the SONY Spresense AI platform. It supports Edge AI and has built-in GPS and hi-res audio. The board is based on the Sony CXD 5602 6-core microcontroller and an FD-SOI processor, which ensure low power consumption of the whole system.
SONY Spresense AI is suitable for solutions that are responsible for analysing and sampling data from sensors using ML and image processing. These are just some of the areas of application of this platform:
- agriculture tech;
- smart cities;
- remote monitoring;
- home automation;
- gesture recognition;
- wildlife monitoring;
- logistics and transport;
- sound diagnostics;
- industrial connectivity.
SONY Spresense AI platform on the SONY website/ Source: sony.com
One of the problems that smart cameras can solve is parking issues in popular tourist cities: cameras with low-cost edge computing solutions can collect data on occupied parking lots and send it to the cloud.
Such a system will run on an SoM or SoC even without a permanent Internet connection, with minimal load on network traffic.
How AI on Edge Can Be Used in Different Industries
We have described some cases of implementing AI on Edge in smart city and urban mobility projects and ADAS software development. But this technology can be applied to almost any sphere to ensure safety and make life easier:
- Medicine and health care: the development of medical instruments and devices based on AI on Edge that enables minimally invasive operations.
- Energy: The implementation of AI on Edge in the development of power electronics will make it possible to analyse data on the state of systems, indicators of sensors and meters, weather conditions, etc. On their basis, it is possible to make forecasts, prevent accidents, and manage resources.
- Production: complex systems of cameras and sensors can provide information about the condition of workers and equipment, simulate scenarios of emergencies, and prevent them. Computer vision is used to control product quality, and Edge AI allows large data sets to be analysed quickly and locally without sending data to the cloud.
The solutions we have described combine AI on Edge, IoT, and ML. This is the basis for building a smart city infrastructure where residents use these technologies daily.
If you have ideas for AI on Edge solutions, write to us, and we will help you implement them.