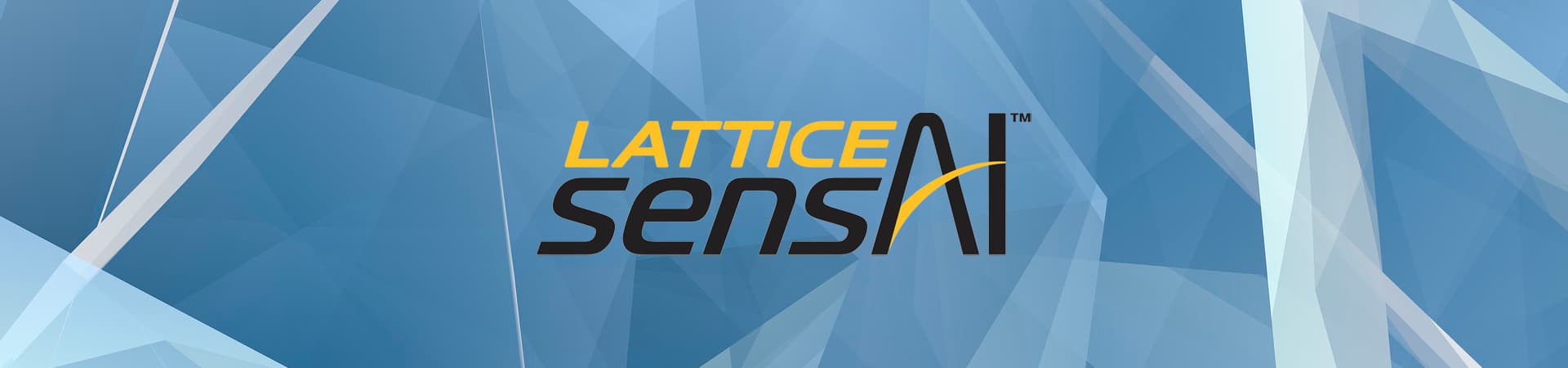
Promwad helps tech companies deploy their AI solutions on edge with Lattice sensAI
We have started to design custom FPGA platforms to help our customers implement their neural networks into low-power and small-scale hardware devices. And Lattice sensAI for Edge AI designs was selected as one of the main frameworks for such projects for original equipment manufacturers (OEM).
The sensAI by Lattice is an advanced tech stack consisting of hardware kits and software tools, neural network IP cores, and reference designs for engineers working on machine learning applications.
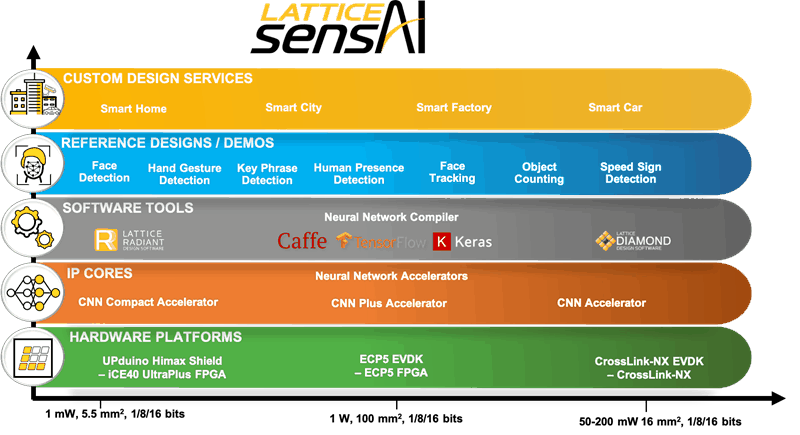
Â
Now, Promwad is using this framework to implement complex AI and ML projects: the company itself is responsible for the hardware design and low-level software, while its clients or partners take on the development of neural networks and machine learning. This collaboration results in complex AI/ML-enabled human-machine interfaces, such as touchless control through speech and gesture, face recognition with identification and object counting, operator compliance in industrial smart cameras, and other FPGA-based applications and AI scenarios tuned to customers' needs.
Power consumption in AI devices varies significantly, depending on the required performance, application, and data model. Some designs may use NVidia Jetson or Xilinx FPGA on the higher end; others employ raw computational power of x86 processors by Intel or AMD. Also, there are some dedicated ASICs targeted at AI acceleration like Intel Movidius or Gyrfalcon.
Â
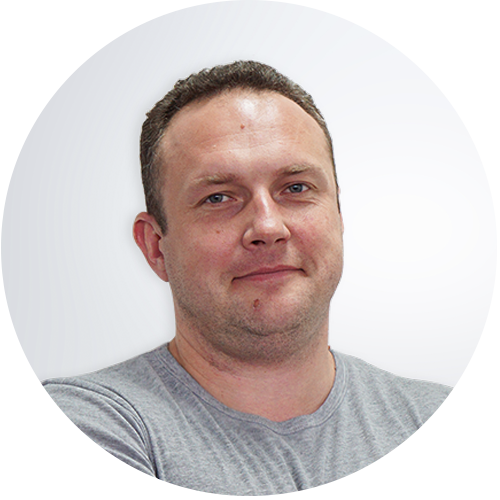
«Typical microcontrollers lack the performance needed for the Edge AI applications, while more advanced solutions like NVidia Jetson are beyond the allotted power budget. Thanks to low-power FPGA, image processing support and sensor-agnostic AI inferencing, the senseAI platform by Lattice is an attractive choice for many low-power Edge AI systems.»Alex Krainov, CTO of Embedded Engineering