Top 10 Hardware Platforms for Embedded AI in 2025
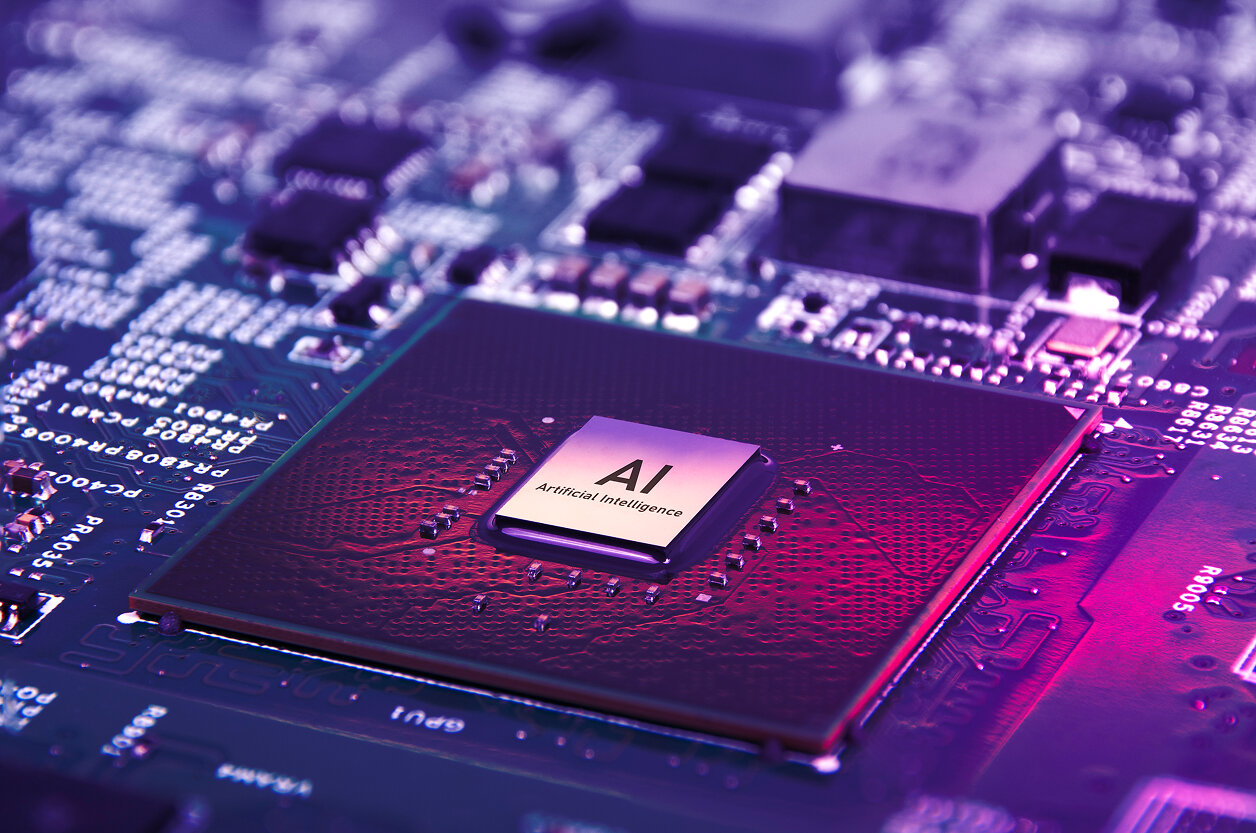
Getting Started: Why Embedded AI Needs the Right Hardware
Embedded AI has become mainstream — powering edge inference in industrial sensors, smart cameras, wearables, autonomous vehicles, and more. But to unlock its full potential, developers need hardware platforms optimized for machine learning tasks under constraints of power, size, and latency.
This article ranks the top 10 embedded AI hardware platforms in 2025 based on performance, ecosystem support, power efficiency, and versatility.
1. NVIDIA Jetson Orin Nano / NX
Why it stands out:
Ampere GPU architecture for high-performance AI inference
TensorRT, CUDA, and deep integration with ROS
Ideal for robotics, drones, vision AI
Key specs:
Up to 100 TOPS
5–15W TDP
Use cases: Industrial robotics, AMRs, smart cameras
2. Google Coral Dev Board (Edge TPU)
Why it stands out:
Ultra-low power AI with dedicated Edge TPU
Optimized for TensorFlow Lite models
Key specs:
4 TOPS at 2W
Use cases: IoT, smart homes, AI sensors
3. Qualcomm QCS6490 / QCS8250
Why it stands out:
AI + connectivity in a power-efficient SoC
Integrated support for computer vision and audio inference
Key specs:
13 TOPS (QCS8250)
5G, Wi-Fi 6E, Bluetooth 5.3
Use cases: Wearables, automotive HMIs, cameras
4. NXP i.MX 93 with Ethos-U65 NPU
Why it stands out:
Secure edge AI with ML-optimized microNPU
Strong support for industrial and automotive
Key specs:
Up to 0.5 TOPS
Arm Cortex-A55 + real-time MCU cores
Use cases: Building automation, energy metering, predictive maintenance
5. Intel Atom x6000E + Movidius Myriad X
Why it stands out:
Powerful x86 CPU combined with a vision-specific neural processor
Key specs:
Up to 1 TOPS (Myriad X)
Industrial-grade x86 compute platform
Use cases: Smart retail, security, industrial gateways
6. Rockchip RK3588 / RK3568
Why it stands out:
Cost-effective AI with integrated NPU
Rich multimedia and display interfaces
Key specs:
Up to 6 TOPS
Up to 8K video encode/decode
Use cases: AI kiosks, media gateways, industrial UI panels
7. Renesas RZ/V2L
Why it stands out:
Dedicated DRP-AI accelerator
Ultra-low power operation and real-time processing
Key specs:
0.5 TOPS at <2W
Cortex-A55 + Cortex-M33
Use cases: Battery-powered cameras, portable AI analyzers
8. Lattice CrossLink-NX with sensAI
Why it stands out:
FPGA-based AI acceleration with ultra-low latency
1W power envelope and small footprint
Key specs:
100 MHz AI pipelines
~1 TOPS equivalent for classification tasks
Use cases: Vision sensors, factory automation, automotive safety
9. Espressif ESP32-S3
Why it stands out:
Low-cost MCU with vector AI acceleration
TensorFlow Lite Micro compatible
Key specs:
Up to 240 MHz dual-core
DSP + AI acceleration instructions
Use cases: AI voice wake, anomaly detection, audio classification
10. Sony IMX500/IMX501 Smart Image Sensors
Why it stands out:
Built-in edge AI inside image sensor itself
Eliminates need for external processors
Key specs:
CNN inference directly on-chip
Event-based output
Use cases: Real-time video analytics, smart cities, presence detection
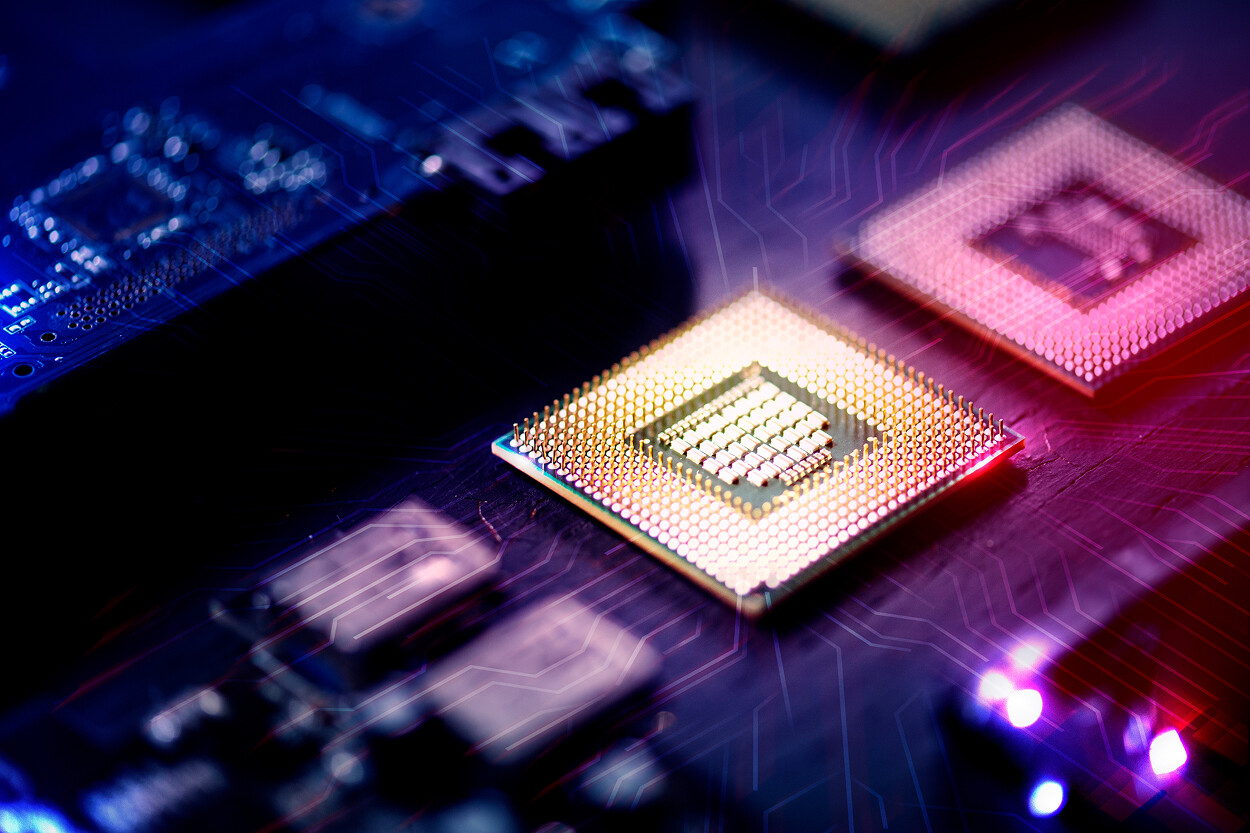
Comparative Table: At a Glance
Platform | AI Performance | Power Use | Target Applications |
NVIDIA Jetson Orin | 100 TOPS | 10–15W | Robotics, vision AI |
Google Coral | 4 TOPS | 2W | IoT, smart sensors |
Qualcomm QCS8250 | 13 TOPS | 5–7W | Cameras, edge analytics |
NXP i.MX 93 | 0.5 TOPS | <3W | Building control, industrial IoT |
Intel + Movidius | 1 TOPS | 6–10W | Retail AI, edge analytics |
Rockchip RK3588 | 6 TOPS | 5–10W | Multimedia AI, kiosks |
Renesas RZ/V2L | 0.5 TOPS | <2W | Smart cameras, portable AI |
Lattice CrossLink-NX | ~1 TOPS equiv | ~1W | Vision sensors, ADAS |
ESP32-S3 | Vector DSP | <1W | Voice, ML inference on microcontrollers |
Sony IMX500 | On-sensor CNN | <1W | Cameras with built-in analytics |
Real-World Example: Building a Smart Traffic Camera with Edge AI
A European smart city startup partnered with Promwad to develop a low-power traffic monitoring camera using on-device image recognition. The goal was to classify vehicle types and detect violations in real time without sending video to the cloud.
Platform Evaluation Criteria:
- Inference latency <50ms
- Power consumption under 5W
- Compact form factor for pole mounting
- LTE connectivity and OTA firmware updates
Promwad’s Recommendation:
Used Rockchip RK3588 for its integrated NPU and multimedia support
Paired with a 4G modem and Promwad-designed OTA module
Deployed YOLOv5 model optimized via TensorRT
Results:
- Achieved 92%+ object detection accuracy in varied lighting
- Device passed thermal and EMC pre-certification
- Reduced bandwidth usage by 80% compared to cloud analytics
Final Thoughts: Matching Your Platform to Your Use Case
There is no one-size-fits-all platform for embedded AI. The right choice depends on your power budget, form factor, model complexity, and deployment environment.
In 2025, we’re seeing greater maturity across AI hardware tiers — from microcontrollers and smart sensors to edge accelerators and embedded SoCs. Successful OEMs will focus on tight integration between AI models, firmware, and silicon.
At Promwad, we help clients evaluate and integrate embedded AI hardware tailored to their use case — optimizing for performance, power, and product lifecycle.
Our Case Studies in Edge AI